News Archive
Best Paper Award at ISDIA 2025
We are delighted to announce that our paper, Self-Supervised Learning for Pre-training Capsule Networks: Overcoming Medical Imaging Dataset Challenges, led by Ms Heba Elshimy, has been awarded the Best Paper Award at ISDIA 2025 , held at the University of Wollongong in Dubai (UOWD) on January 3-4, 2025.
Abstract: Deep learning in medical imaging faces a critical challenge: the scarcity of large, high-quality annotated datasets. While pre-training on extensive datasets is a common solution, it demands substantial computational resources that aren't always available to researchers. Our work demonstrates how to overcome these limitations by improving Capsule Networks (CapsNet)—an innovative but under-utilised architecture—for polyp classification using limited, imbalanced data.
We developed a novel approach using self-supervised learning (SSL), where CapsNet creates its own training signals through tasks like image colourisation, contrastive learning, and in-painting. This method achieved improved performance on downstream tasks, increasing the classification accuracy by 5.26% compared to other weight initialisation methods. This work opens new paths for deep learning in the medical imaging field, particularly when working with challenging datasets.
Published: January 2025
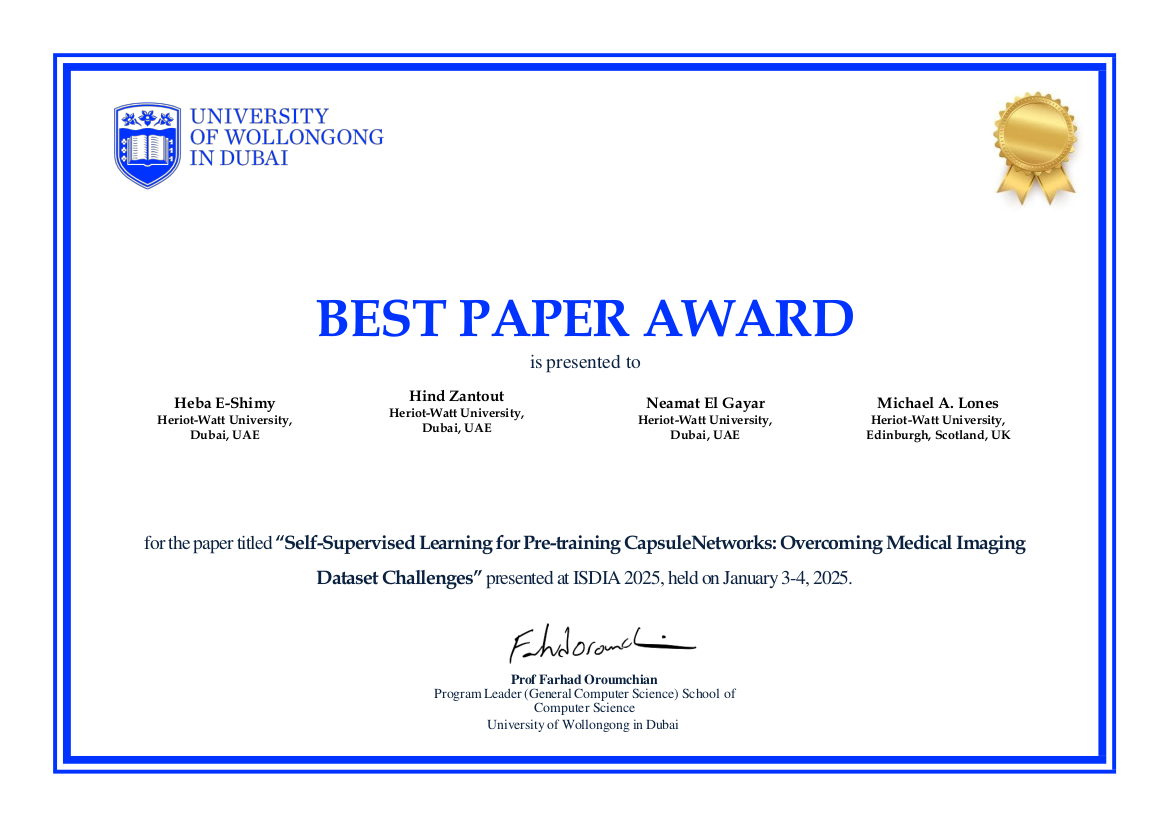